All published articles of this journal are available on ScienceDirect.
A 16 Epithelia-mesenchymal Transition Associated LncRNAs Signature to Optimize Prognosis Predication of Stomach Adenocarcinoma
Abstract
Aim:
The study aimed to identify critical long non-coding RNAs (lncRNAs) and constructed a prognostic signature to optimize prognosis predication of patients with Stomach Adenocarcinoma (STAD).
Background:
STAD is a common malignant tumor with a high metastasis rate and low survival rate. LncRNAs participate in the regulation process of epithelial-mesenchymal transition (EMT) and the development of STAD.
Methods:
RNAseq data were obtained from TCGA-STAD, while 200 EMT-associated genes (EAGs) from the ‘HALLMARK_EPITHELIAL_MESENCHYMA-L _TRANSITION’ gene set. Differentially expressed EAGs and EMT-associated lncRNAs (EALs) were identified. Moreover, Lasso-Cox regression analysis was used to construct a signature of differentially expressed EALs, and univariate and multivariate analyses, Kaplan-Meier analysis, receiver operating characteristic curve (ROC) analysis, and nomogram were conducted to predict its prognostic value. An enrichment functional analysis was performed. Quantitative Real-Time PCR (qRT-PCR) was used to determine lncRNAs expressions in cell lines.
Results:
A total of 52 differentially expressed EAGs and 320 EALs were identified in this study. Meanwhile, 16 EALs were used to construct the signature, and further analysis indicated that it had a high prognostic value for STAD patients. Enrichment functional analysis revealed the signature was correlated to tumor immunity in STAD. Moreover, three novel EALs expressions were confirmed in cell lines.
Conclusion:
A novel survival signature was established to predict and evaluate the prognosis of STAD patients.
1. INTRODUCTION
There were an estimated 1,089,103 new cases of gastric carcinoma (GC) and 768,793 cancer deaths worldwide in 2020 [1]. Accounting for over 95% of GC [2], stomach adenocarcinoma (STAD) aggravates the medical burden because of its high metastasis rate and low survival rate [3]. Due to the different prognoses of patients in the same stage, there is an urgent need for determining new biomarkers to predict the prognosis of these patients.
Epithelial-mesenchymal transition (EMT), a continuum of biological processes that enhances anti-apoptosis, and invasive and migratory abilities in cells, is now considered to be highly relevant to tumor progression and metastatic events [4]. Helicobacter pylori (H. pylori), the main risk factor of GC [5], is known to up-regulate the expression of zinc finger E-box-binding homeobox 1 (ZEB1) expression by activating NF-κB, subsequently initiates mesenchymal transition in gastric epithelial cells and contributes to the occurrence of cancer [6].
Long non-coding RNA (lncRNA) is a type of regulatory transcript with diverse sizes of around 200 nucleotides or more in length [7]. Dysregulation of lncRNA brings about cellular function disorder, abnormality of cell proliferation, promotion of invasion and metastasis, and induction of angiogenesis [8]. Moreover, several lncRNAs have been reported to be involved in cell proliferation, invasion, and migration via EMT in GC [9, 10].
Therefore, we hypothesized that EMT-associated lncRNAs (EALs) played a significant role in the development of STAD. In this study, we collected EAGs and identified the expression status, based on the RNAseq data from The Cancer Genome Atlas (TCGA) database, and established a survival signature to optimize the prognostic prediction of STAD patients. Then, we carried out gene enrichment analysis (GSEA) to explore the potential mechanisms and qRT-PCR was used to determine lncRNAs expressions in cell lines.
2. METHODS
2.1. Data Acquisition and Analysis
The RNAseq data and corresponding clinical information of patients with STAD were obtained from the TCGA database (https://portal.gdc.cancer.gov/). In this study, 200 EMT-associated genes (EAGs) were obtained from the ‘HALLMARK_EPITHELIAL_MESENCHYMA-L_TRANSITION’ gene set based on Molecular Signatures Database (MSigDB) v7.4. Firstly, differentially expressed EAGs were identified by the “limma” R package. Then, Pearson correlation analysis between EAGs and EALs was conducted to determine the EALs based on the correlation coefficient (|Corpearson|>0.4) and P<0.001.
2.2. Construction of Differentially Expressed EALs Signature
A univariate Cox regression analysis was conducted to find prognostic EALs of STAD (p<0.05). Subsequently, the least absolute shrinkage and selection operator (LASSO) Cox regression algorithm was performed to identify EALs with powerful prognostic significance and build a prognostic signature model. The coefficients of the EALs were calculated through the “glmnet” R package. The risk score was calculated according to expression value and coefficients of lncRNAs: Coefficient lncRNA1 * lncRNA1 expression) + (Coefficient lncRNA2 * lncRNA2 expression) + ⋯ + (Coefficient lncRNAn * lncRNAn expression). Meanwhile, univariate and multivariate analyses were used to screen whether lncRNA risk signature can be an independent factor. Besides, Kaplan-Meier analysis to explore overall survival (OS) between high- and low-risk groups, and receiver operating characteristic curve (ROC) analysis was constructed to evaluate the prognostic value of the signature [11].
2.3. Enrichment Functional Analysis
Kyoto Encyclopedia of Genes and Genomes (KEGG) and Gene Ontology (GO) analysis using R packages were conducted to find the potential function of EMT-associated differentially expressed mRNAs. Besides, gene set enrichment analysis (GSEA) was performed to identify the significant pathway enriched in the EALs risk signature, with P<0.05 and FDR<0.05 as statistical differences. Furthermore, single sample gene set enrichment analysis (ssGSEA) was applied to quantify the enrichment levels of 16 immune cells and assess the activity of 13 immune-associated pathways. The flow chart of analysis was shown in Fig. (1).
2.4. Cell Cultures
STAD cell lines MGC-803 and human normal gastric epithelial cell line GES-1 were purchased from the American Type Culture Collection (ATCC, Manassas, VA, USA). Both cells were cultured in RPMI-1640 Medium (Life Technologies, Grand Island, NY, USA) supplemented with 10% fetal bovine serum (FBS, Life Technologies) and antibiotics (100 units/ml penicillin and 100 ug/ml streptomycin) at 37°C, 5% CO2 in a humidified atmosphere incubator.
2.5. Quantitative Real-time Polymerase Chain Reactions (qRT-PCR)
The total RNA of cell lines was extracted with TRIzol reagent (Invitrogen, China) according to the manufacturer's instructions. Using the PrimeScript RT Reagent Kit (Takara. China), the RNA was reversely transcribed into cDNA, and quantitative real-time PCR (qRT-PCR) analyses were quantified with SYBR Green (Takara, China). The expression of EALs was calculated based on the 2−ΔΔCt method with GAPDH as an internal reference. And, qRT-PCR primers used in this study were as follows: AC245041.2 forward primer,5’- GAGATGGCCTGGTGGAAA -3’; reverse primer, 5’- ACCTGGCTTCGGTCACTC - 3’; LINC02544 forward primer, 5’- GGTTGTTCTCATTCGTGGCT -3’; reverse primer, 5’- AGGCGGTTTCAGTGTTGG -3’; AP001528.2 forward primer,5’- AGGGAGGAGGATGGAAGG -3’; reverse primer, 5’- GTGCAGGTTTGTTACGTGAGTA - 3’; GAPDH forward primer, 5’- GGACCTGACCTGCCGTCTAG- 3’;GAPDH reverse primer:3’-GTAGCCCAGGATGCCCTT GA- 5’.
2.6. Statistical Analysis
All statistical data were analyzed using R version 4.0.2. The Wilcoxon test (Mann-Whitney test) and Fisher’s exact test were applied to evaluate continuous variables and categorical data, respectively. For each statistical analysis, a P-value less than 0.05 was considered statistically different.
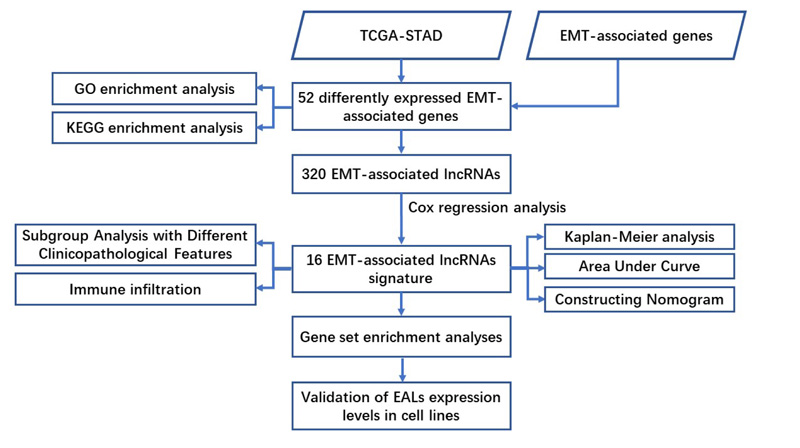
3. RESULTS
3.1. Identifying Differentially Expressed Genes and Enrichment Analysis
We identified 52 differentially expressed EMT-associated genes (24 upregulated and 28 downregulated) (Fig. 2A, B). Meanwhile, an enrichment analysis of these 52 genes was conducted. Biological processes (BP) were mainly enriched in the extracellular matrix organization, extracellular structure organization, and cell-substrate adhesion. Cellular components (CC) were mainly enriched in the collagen-containing extracellular matrix, extracellular matrix component, and collagen trimer. Molecular functions (MF) were mainly enriched in extracellular matrix structural constituent, Actin binding. KEGG is mainly enriched in focal adhesion, IL-17 signaling pathway, NF-κB signaling pathway, PI3K-Akt signaling pathway, and epithelial cell signaling in Helicobacter pylori infection (Fig. 2C, D).
3.2. Constructing the Prognostic EALs Signature
A total of 320 EALs was identified from the correlation analysis. Meanwhile, 19 candidate EALs that highly correlated with OS were selected by univariate Cox regression analysis (P <0.05) (Fig. 3A) (AC245041.2, BOLA3-AS1, AC011451.1, POLH-AS1, AL161785.1, SERPINB9P1, LINC01614, MAGI2-AS3, AP001528.2, AC010503.4, LINC02542, LINC2544, LINC02526, AL353804.1, PVT1, AC037198.1, AP000695.2, RRN3P2, LINC01094). Subsequently, we established an EMT-associated lncRNAs signature with 16 EMT-associated lncRNAs through lasso Cox regression analysis, which was divided into high- and low-risk groups on the basis of median risk score. The risk score for each STAD patient was calculated according to the risk formula: Riskscore= AC245041.2 *(0.174084699887985)+ BOLA3.AS1* (0.242017960315735) + AC011451.1 * (-0.514353798961673) + AL161785.1 *(0.336108363159172) + LINC01614 * (0.0044176080712068) + MAGI2.AS3 * (0.00922891591580578) + AP001528.2 * (0.078616299 2015131) + AC010503.4 * (-0.0475079462899831) + LINC02542 * (0.000266198370952843) + LINC02544 * 0.0536404575234678 + LINC02526 * (0.463622058746716) + AL353804.1+(-0.810590348346779) + PVT1*(-0.32637153 4805745) + AC037198.1* (0.0965119232406708) + AP000695.2*(0.348610133333211) + RRN3P2 * (0.657044 309706091). Besides, correlations between the levels of these 16 EALs and EMT genes are shown in Fig. (3B).
3.3. Prognostic value of the EALs Signature in STAD
According to the patient’s risk curve, the patients were divided into high- and low-risk groups based on the median risk score, which was approximately -0.61 (Fig. 4A). The patient’s risk survival status plot indicated that the higher the patient’s risk score, the shorter survival time and the more dead patients with STAD (Fig. 4B), and the 16 prognostic EALs expressions were shown as a heat map (Fig. 4C). The results of the Kaplan-Meier analysis showed the expression of high-risk lncRNA signatures correlated with poorer survival (P=3.87e-08, Fig. 5A). And an area under the curve (AUC) of the lncRNAs signature was 0.695, which is higher than the other clinicopathological features, indicating high performance in predicting the prognosis of STAD (Fig. 5B). To determine whether the risk score was an independent prognostic factor, Univariate and multivariate COX analysis was performed, and the results revealed that risk score had a close relationship with OS of STAD patients (Univariate: hazard ratio (HR) = 3.519, 95% CI = 2.560-4.838, P< 0.001; Multivariate: HR = 3.722, 95% confidence interval(CI) = 2.664-5.200, P< 0.001 (Fig. 5C, D), which suggested that the risk score could be an independent valuable prognostic factor in patients with STAD.
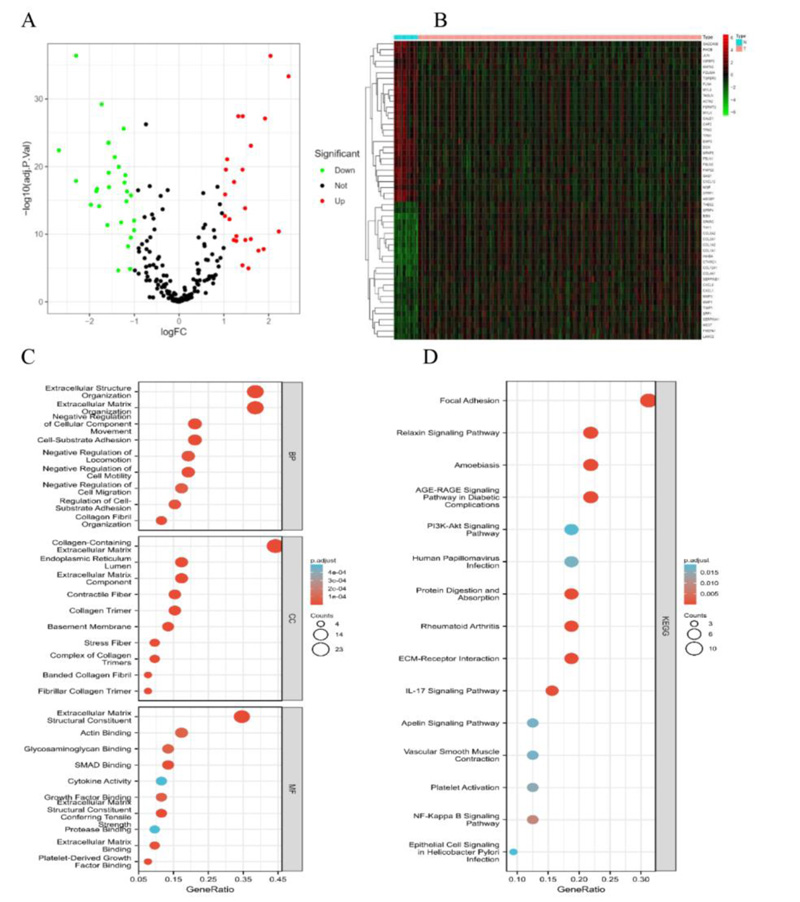
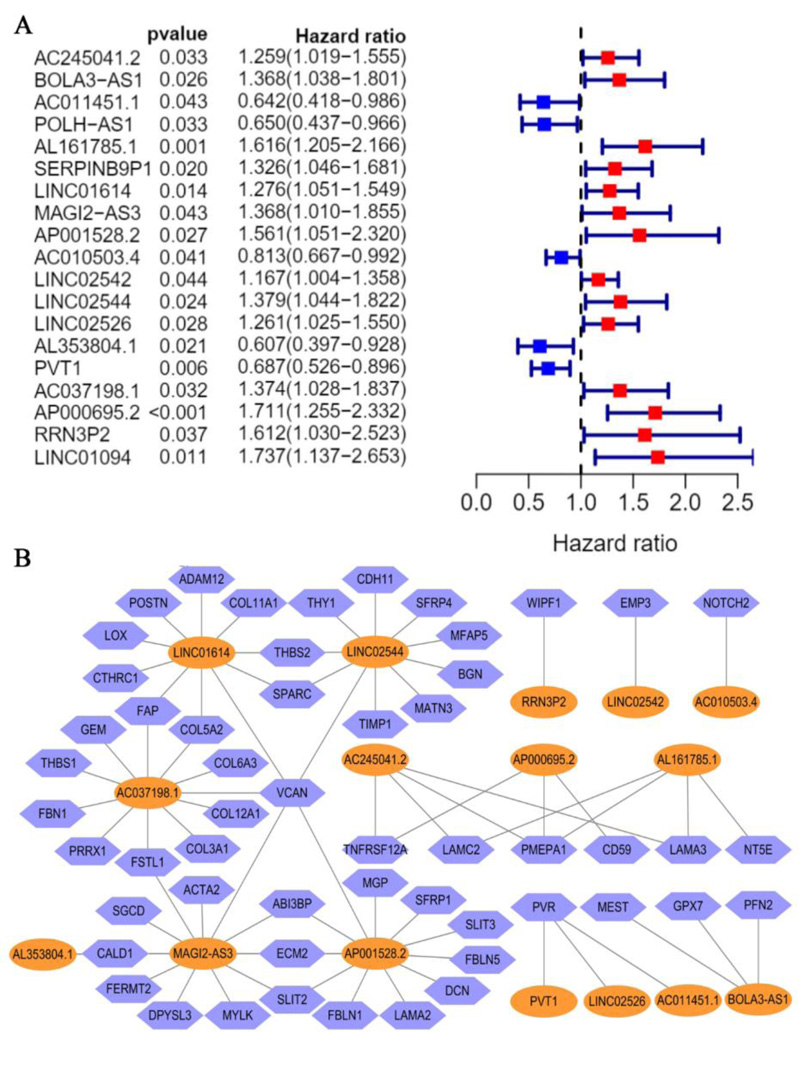
3.4. Constructing Nomogram of STAD
Moreover, we constructed a nomogram model by combining clinicopathological features and novel EALs signature for STAD, which may be applied in the management of patients with STAD by physicians (Fig. 6).
3.5. Subgroup Analysis with Different Clinicopathological Features and Immune Landscape
Furthermore, stratifying subgroups by age(age > 65 and age ≤ 65), grade(G1-2 and G3), stage(I-II and III-IV), T classification(T1-2 and T3-4), M classification(M1 and M0), N classification(N0 and N1-3), we investigated the prognostic value of the signature in subgroups of patients with STAD with different clinical features. The analysis showed that the OS of STAD patients in the high-risk group was shorter than low-risk group, according to age(P<0.001 in age > 65 and P=0.008 in age ≤ 65), grade(P=0.046 in G1-2 and P<0.001 in G3), stage(P=0.008 in I-II and P<0.001 in III/IV), T classification(P=0.047 in T1-2 and P<0.001 in T3-4), M classification(P=0.028 in M1 and P<0.001 in M0), N classification(P=0.023 in N0 and P<0.001 in N1-3) (Fig. 7).
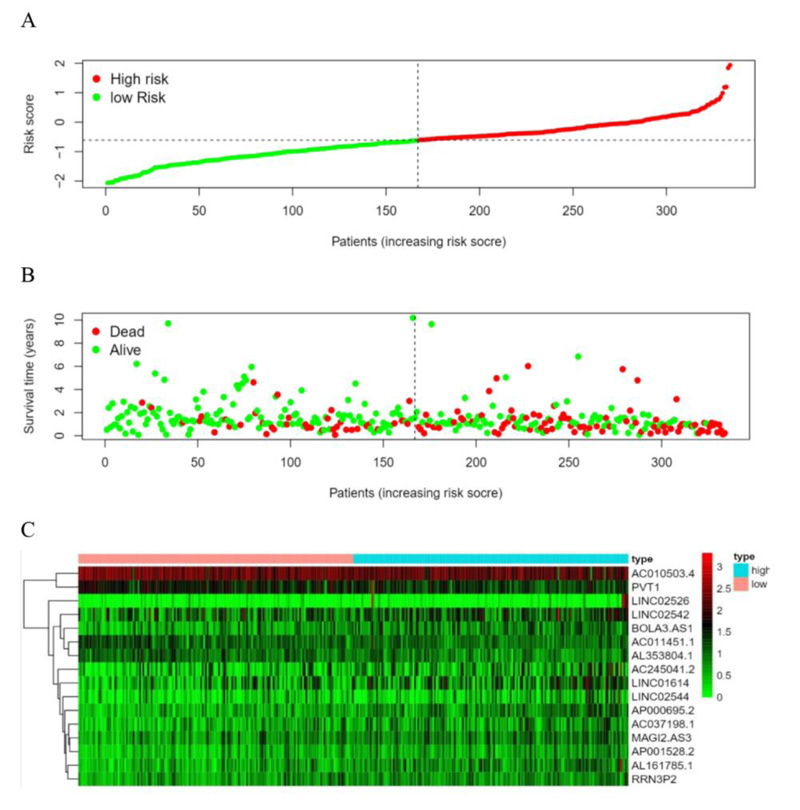
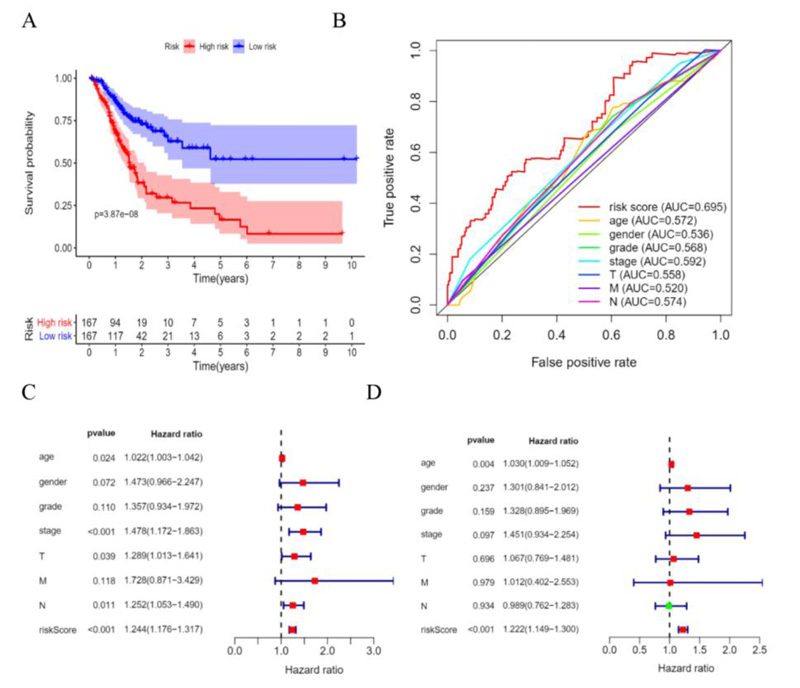
Previous researches have revealed that EMT had close interaction with infiltrated immune cells in the tumour microenvironment [12]. Therefore, we intended to examine the relationship between the signature and immune cells by performing a ssGSEA analysis. The results suggested that the scores of aDCs, DCs, iDCs, macrophages cells, Tfh cells, Th1 cells, Th2 cells, and Treg cells were also significantly higher in high-risk groups than those in low-risk groups (Fig. 8A). Besides, the scores of APC co-inhibition/co-stimulation, CCR, checkpoint, HLA, MHC class I, para-inflammation, and T cell co-inhibition/co-stimulation were significantly higher in the high-risk group, however, type I/II IFN response was significantly higher in the low-risk group (Fig. 8B).
3.6. Gene Set Enrichment Analyses
The results of GSEA indicated that the high-risk groups were mainly enriched in EMT, inflammatory response, apical junction, complement, TNF signaling via NF-B, angiogenesis, and other tumor-related pathways (Fig. 9).
3.7. Validation of EALs Expression Levels in Cell Lines
Finally, we examined the expression of 16 lncRNAs in TCGA-STAD and found that PVT1, AC245041.2, LINC01614, LINC02544, AP000695.2 were significantly upregulated and AP001528.2 were significantly downregulated in STAD tissues (P<0.001, (Fig. 10A, B), indicating they were novel oncogenes and antioncogene. It has been reported that lncRNA PVT1 [13], LINC01614 [14], and AP000695.2 [15] were upregulated and correlated with poor prognosis in GC. However, there was no study on AC245041.2, LINC02544, and AP001528.2 in STAD and their expression needs to be identified. Hence, we performed confirmation of GES-1 and MGC-803 by qRT-PCR. Consistently, compared with GSE-1, the expression levels of AC245041.2, and LINC02544 in MGC-803 were remarkably higher (Fig. 10C, D), while AP001528.2 was significantly lower downregulated (Fig. 10E). Moreover, KM plotter analysis indicated that AC245041.2, LINC02544, and AP001528.2 were correlated with poor prognosis (Fig. 10F-H).
4. DISCUSSION
Cancer prognosis prediction is important for both patients and clinicians, for the reason that it helps to enhance health education and lifestyle intervention, improve the management of prognosis, moreover, replace outcome assessment indexes [16, 17]. EMT is implicated in cellular migration [18], invasion, anti-apoptosis, metabolism [19], and immunosuppression [20] and drug resistance [21]. Meanwhile, it has been proven to play a crucial role in STAD development from tumorigenesis to metastasis and has a close connection with poor prognosis [22]. LncRNAs are involved in the modulation of EMT processes [23], so identifying critical lncRNAs and figuring out their molecular mechanisms of EMT in STAD are of great significance to control tumor progression.
Herein, we identified 52 differentially expressed EAGs in the TCGA-STAD cohort, and the results of GO and KEGG analysis showed that they were enriched in tumor-related pathways. Meanwhile, we identified 19 prognostic-related EALs (AC245041.2, BOLA3-AS1, AC011451.1, POLH-AS1, AL161785.1, SERPINB9P1, LINC01614, MAGI2-AS3, AP001528.2, AC010503.4, LINC02542, LINC2544, LINC02526, AL353804.1, PVT1, AC037198.1, AP000695.2, RRN3P2, LINC01094) as independent prognostic factors for STAD. Then, with lasso Cox analysis, a novel prognostic signature based on 16 EALs was constructed, and the risk assessment model demonstrated higher specificity and sensitivity in predicting the survival of STAD patients compared to traditional clinicopathological features. In addition, with higher levels of immune cell infiltration and immune function activation by ssGSEA, the high-risk group was enriched in immune-related pathways through GSEA. The results suggested that aDCs, DCs, iDCs, macrophages cells, Tfh cells, Th1 cells, Th2 cells, and Treg cells were related to the prognosis of STAD patients, and immune-related function including APC co-inhibition/co-stimulation, CCR, checkpoint, HLA, MHC class I, para-inflammation, T cell co-inhibition/co-stimulation and type I/II IFN response were significantly different in two groups. Some cytokines and chemokines, such as IL-17 [24], IL-6 [25], IL-33 [26], IL-23 [27], and TGF-β [28], released by immune cells, including macrophages, dendritic cells, T cells, etc, can induce EMT and cause the development and metastasis of carcinoma. It has been reported that M2 macrophage polarization can enhance the processes of EMT and metastasis in GC [29].
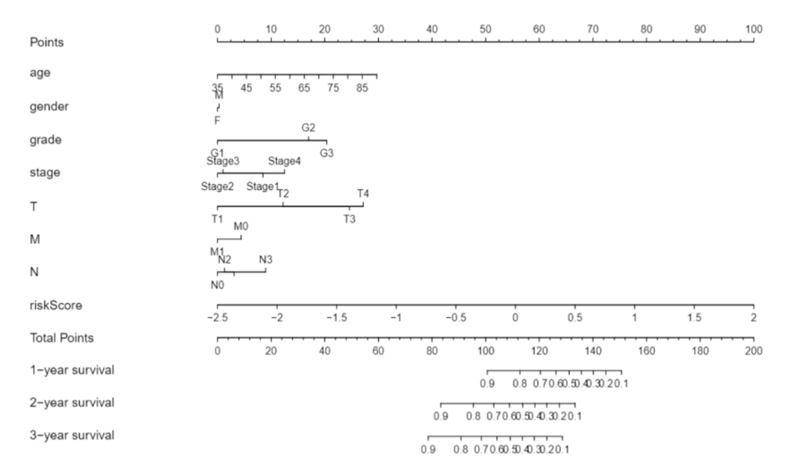
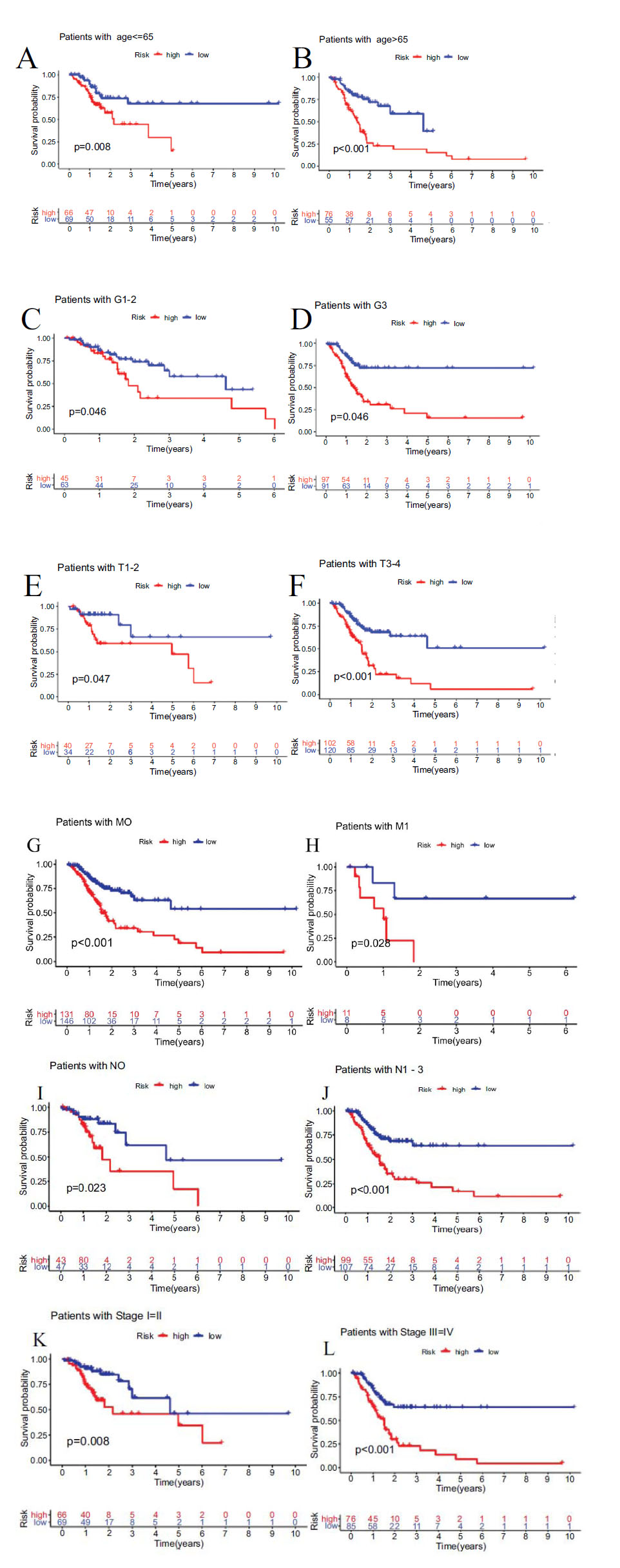
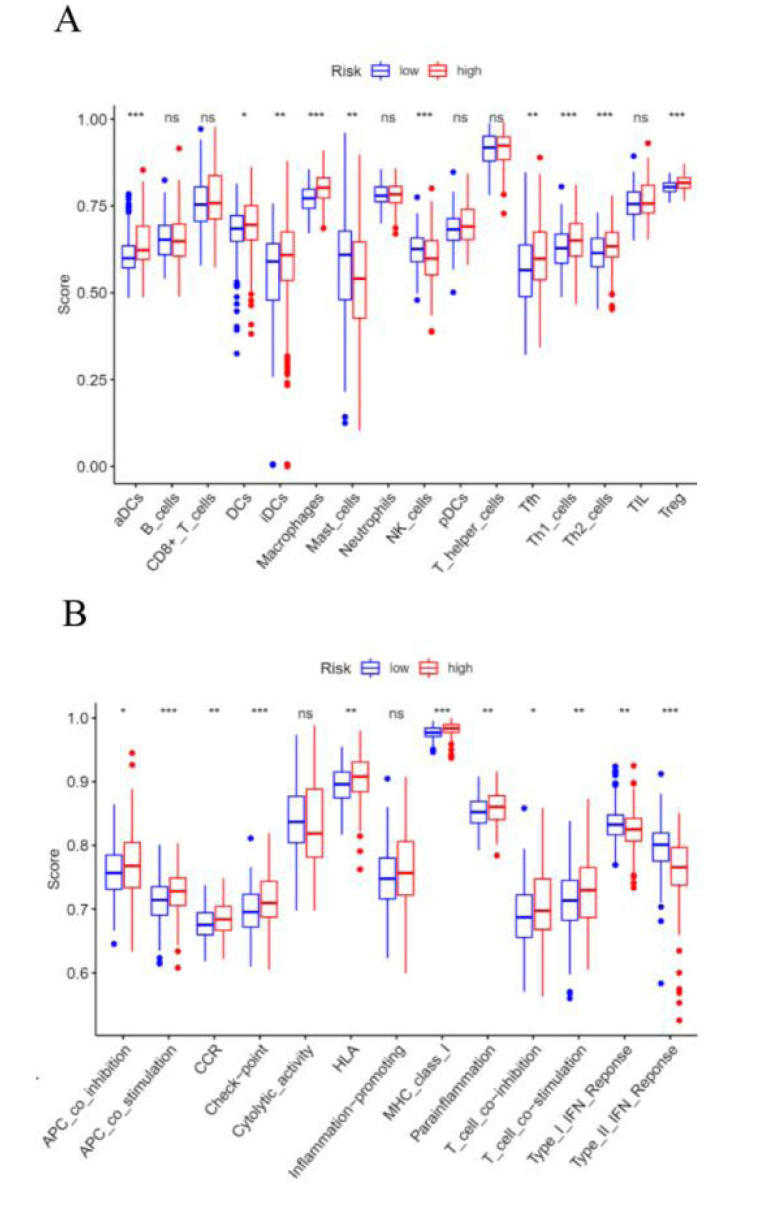

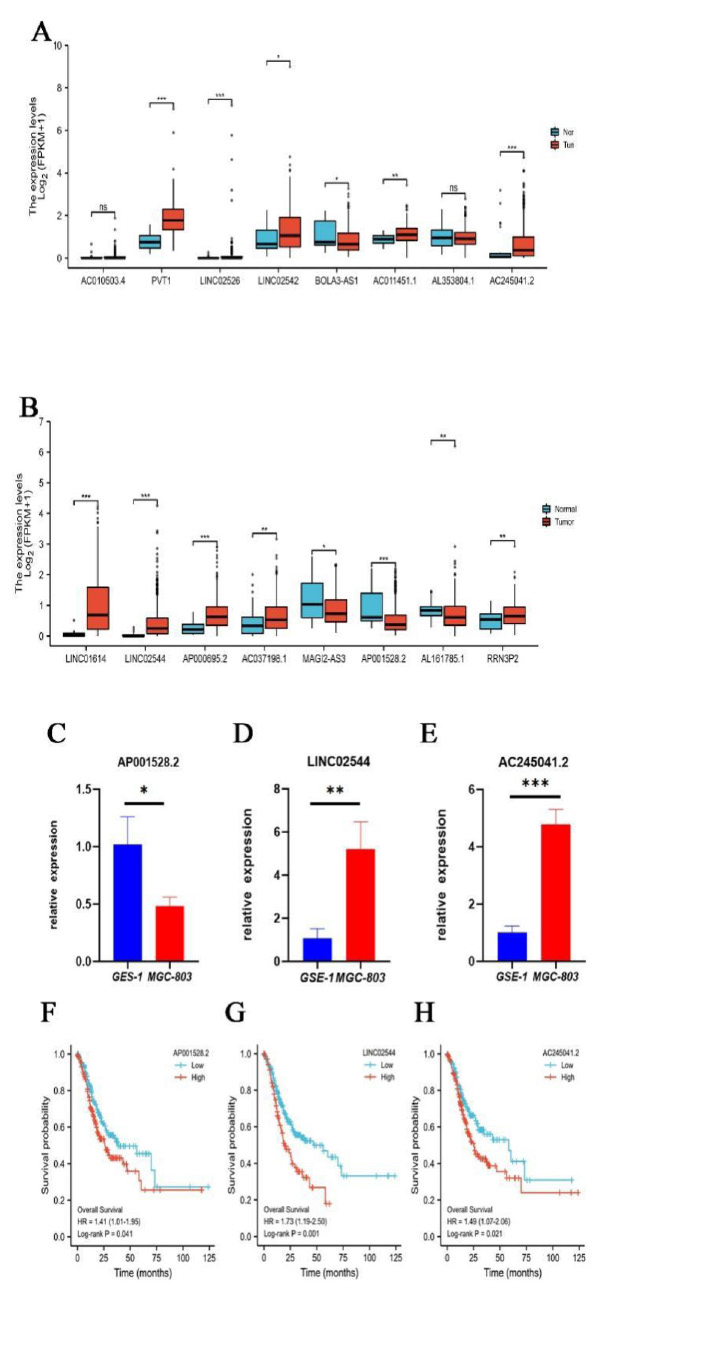
Within these EALs, the correlation between some lncRNAs and STAD had been reported. AP000695.2 is linked to the TNM stage (specially T stage and distant metastasis) of STAD and is involved in tumor-related pathways in cancer [15]. A recent study found that ectopic expression of lncRNA MAGI2-AS3 is positively controlled by BRD4, increasing the expression of ZEB1 through sponging miR-141/200a-3p, leading to poor prognosis of STAD [30]. LINC01614 has been shown to be associated with the proliferation and migration of STAD, which had been validated by overexpression and CRISPR-Cas9 knockout experiments [14]. LncRNA PVT1 induces EMT and facilitates aggressive vasculogenic mimicry formation via the activity of the STAT3/Slug axis [13].
There are certain correlations between lncRNAs, EMT, and STAD. For instance, a prostate transmembrane protein, androgen induced 1 (PMEPA1), could be stimulated by activation of TGF-β, a crucial promoter of tumor metastasis by inducing EMT to improve the metastatic ability of tumor cells. The expression of PMEPA1 mRNA in gastric tumor tissues was significantly higher compared with surrounding normal tissues [31], while reducing PMEPA1 expression by gene knockdown can enhance TGF-β/Smad signaling, suppress cell proliferation, and tumorigenic potential in different types of cancer [32-34]. As shown in Fig. (3B), 3 EALs were identified to have positive correlations with PMEPA1, including AP000695.2, AC245041.2, and AL161785.1. And, it has been reported that AP000695.2 was linked to the TNM stage (specially T stage and distant metastasis) of STAD and involved in tumor-related pathways in cancer [15]. Overexpression of VCAN facilitated cell cycle progression and cell proliferation by upregulating Cyclin D1 and Cyclin E, crucial markers of EMT, and promoted cell migration and invasion by negatively regulating Vimentin and N-Cadherin, a cancer suppressor. However, converse consequences occurred after the knockdown of VCAN in GC cells, the cell cycle was induced to arrest at G0/G1 phase and malignant transformation as well as metastatic tumor progression was inhibited [35]. VCAN was found co-expressing to 5 EALs, involving lncRNA MAGI2-AS3, LINC01614, LINC02544, AC037198.1, and AP001528.2. Among these EALs, lncRNA MAGI2-AS3 and LINC01614 have been experimentally validated to be associated with the proliferation and migration of STAD [14]. In addition, to our knowledge, we confirmed the expression levels and conducted survival analysis of AC245041.2, LINC02544, and AP001528.2, which were most differentially expressed and hadn’t been reported.
However, there are some limitations in this study. Some biases are likely to exist due to data from public databases. And more experience should be conducted to provide strong support for this result, such as lncRNAs for a phenotype of knock-in/down or overexpressing cells. Besides, some lncRNAs have not been reported and their functions remain unclear, which needs to be further explored.
CONCLUSION
In summary, this study constructed a prognostic signature comprising 16 EMT-associated lncRNAs in STAD, which had powerful value in predicting the OS of patients with STAD, clinicopathological factors, and immune infiltration. Moreover, the biological function and pathways of the EALs were identified. Finally, three novel EALs expressions, AC245041.2, LINC02544, and AP001528.2, were confirmed in the cell line. This study provides a valuable signature to improve the prognostic prediction for patients with STAD.
LIST OF ABBREVIATIONS
(lncRNAs) | = Identify Critical Long non-coding RNAs |
STAD | = Stomach Adenocarcinoma |
EMT | = Epithelialmesenchymal Transition |
GC | = Gastric Carcinoma |
OS | = Overall Survival |
AUTHORS' CONTRIBUTIONS
Conception and design: X Chen; Collection and assembly of data: Y Chen, Y Huang, X Jiang, J Zheng; Data analysis and Manuscript writing: Y Yan, X He.
ETHICS APPROVAL AND CONSENT TO PARTICIPATE
Not applicable.
HUMAN AND ANIMAL RIGHTS
No animals/humans were used for studies that are the basis of this research.
CONSENT FOR PUBLICATION
Not applicable.
STANDARDS OF REPORTING
The authors have completed the TRIPOD reporting checklist.
AVAILABILITY OF DATA AND MATERIALS
All data analyzed during this study are available from the corresponding author [X.C] upon reasonable request.
FUNDING
This work was supported by the Natural Science Foundation of China, No.81904145; Natural Science Foundation of Guangdong Province, China (2019), No.2019A1515011145; Major research project of Guangzhou University of Chinese medicine, China, No. A1-AFD018201A51; The first affiliated hospital of Guangzhou University of Chinese medicine “Innovative Strong Hospital ” clinical research project, China (2019), No.2019IIT19; Liu Fengbin, Guangdong famous traditional Chinese medicine inheritance studio (Guangdong traditional Chinese medicine office [2020] no.1).
CONFLICT OF INTEREST
The authors declare that the research was conducted in the absence of any commercial or financial relationships that could be construed as a potential conflict of interest.
ACKNOWLEDGEMENTS
The authors are grateful to Lingnan Medical Research Center for their experiment support.